Data Center Optimization
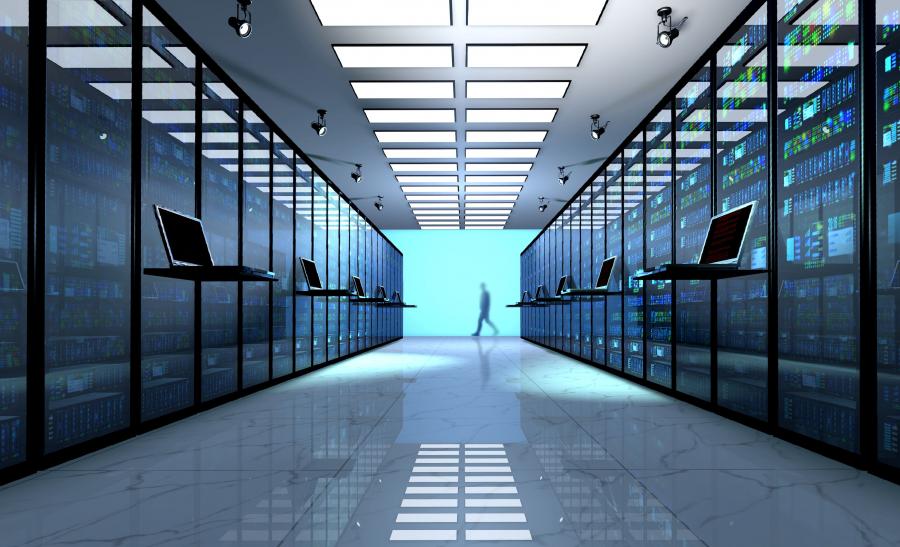
Introduction
Data Center Optimization refers to any series of processes and policies that are implemented to increase the efficiency of data center operations without sacrificing functionality or performance. It is a method of enhancing the overall performance of a business's data center capabilities through the implementation of various IT strategies such as server consolidation, server virtualization and service-oriented architecture (SOA) to increase processing efficiency, network availability and business scalability.
Server consolidation helps by minimizing the number of active servers a data center employs. Often, data centers have many under-utilized servers. The processes these servers perform can usually be consolidated into fewer servers.
With this strategy, a company can save a lot of money because it will have less infrastructure costs, less maintaining costs and less running costs (because as it will have less servers, it will reduce its power consumption).
How to measure optimization
At first, optimization might sound like a very subjective or abstract concept, but it is not. An optimization strategy’s effectiveness is measured by the facility’s Power Usage Effectiveness (PUE) score, which compares the total power requirements of computing equipment against the data center’s total power consumption. That being said, if we compare two data centers, the one with the lower PUE score is the most optimal.
As we mentioned previously, lower power usage equals to lower costs, so if two data centers process the same amount of data, the one with fewer power usage will be the one with more revenue, because it will be the one with fewer costs (assuming they both charge the same for their services).
Optimization strategy
The optimization of a data center goes through some steps, which are the following ones:
- Collecting information: about network utilization, server power consumption, and cooling performance. This is very important because it will establish the baseline for measuring the efficiency of a data center’s infrastructure. With a good set of information, we can start to create strategies such as shifting compute workloads, powering down servers when they are not needed, and cycling up resources during high demand periods, which can greatly improve energy efficiency without compromising performance.
- Buying ahead: an important part of the optimization process is preparing for scalation. That being said, a common error when purchasing equipment is buying equipment that only meets their actual and existing needs. If the data center grows, this equipment can become a bottleneck, so it is best if we buy ahead for equipment needs. This way, not only our hardware will not be running at its full power, which can be bad, but also if we need to expand in the future, we will not have as many problems as we would have had with less powerful equipment. Moreover, running a single, high-density server cabinet, for example, is generally more efficient than running the same processing load across multiple low-density cabinets. So, purchasing better equipment will result on a higher inversion but will result in an important upgrade in terms of scalability.
- Equipment deployment: a poor server deployment could introduce latency into network systems. A wrong deployment could increase cooling costs if servers are unable to vent heat effectively or difficult maintaining if there is a bad cable management, for example.
- Optimization: as it was previously explained, optimization can deliver a big number of performance benefits. The optimization mainly focuses the following:
- Better compute usage: through server virtualization or hybrid cloud deployments. This helps to manage computing workloads more effectively.
- Better cooling efficiency: cooling energy represents about 40% of the total energy consumed by a data center, so it is very important to lower the cooling costs.
- Better uptime reliability: optimized datacenters tend to be more reliable since they use energy more effectively. This can be achieved because the cooling system and the machines are working at their optimal point and because of network redundancy to avoid single point of failure. Moreover, since we have managing tools that collect information, we can troubleshoot problems more easily and maintain a very high level of network uptime.
Future of data center optimization
Recent researches state that the complexity of the data center scenarios that start emerging is going to be extremely high.
In the past, heuristics have been used as workarounds to address hard problems with variable results. The major problem with such heuristics is that they need to make generic assumptions on some workload, user or hardware characteristics that may hardly adapt to changing conditions.
Even though heuristics have worked well in the past, the future will require a more sophisticated solution. This is the reason why there are starting to appear self-learning algorithms, which are based on deep learning techniques, which main goal is to manage data centers to optimize them to their best point and help manage and collect information about their usage.
Authors
Joan Farràs
Ferran Montoliu