Calorimeter Reconstruction Innovations for the LHCb Experiment
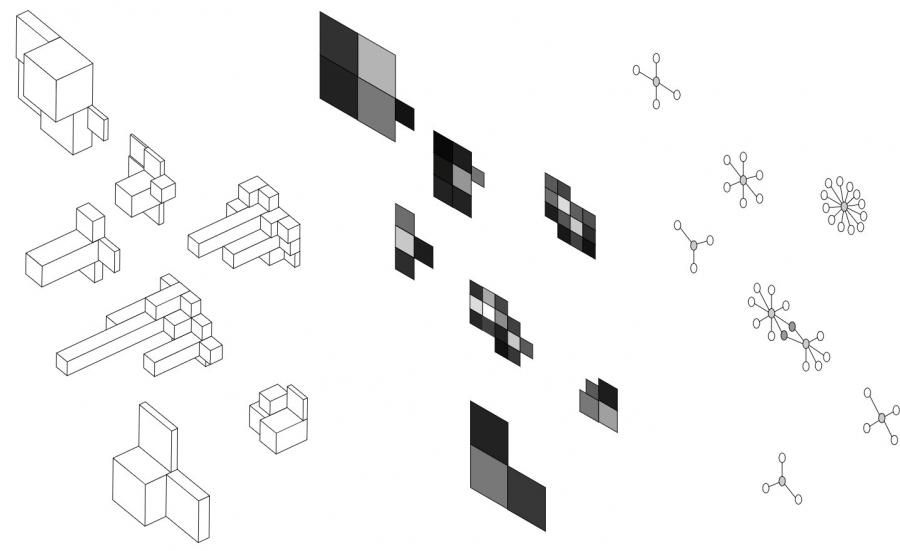
This thesis focuses on software contributions to the LHCb experiment at CERN, specifically for the Calorimeter system in LHCb Upgrade I context. The main contributions concern the study of alternative algorithms for calorimeter data reconstruction. The first approach employs a segmented deep learning technique, breaking down the reconstruction problem into steps learned by small convolutional neural networks. Although promising, it lacked an efficient inference engine inside the LHCb framework. The second approach presents a graph-based clustering algorithm, showing equivalent cluster resolution to the LHCb's existing method, but with higher efficiency and significantly improved execution time, which is now the default solution for calorimeter reconstruction in the Run 3 period. This work also comprises a first approach to improve the current calorimeter reconstruction algorithm in the GPU Allen framework for HLT1, while the other approaches aim to the CPU reconstruction sequence in HLT2.
Additionally, the thesis addresses part of the calorimeter commissioning for Run 3, detailing the time alignment task for the Electromagnetic and Hadronic calorimeters. This involves the adaptation of the previous method to new electronics, gathering and analyzing data, and providing fine time alignment for the around 10,000 calorimeter channels within 1 ns precision.
Add new comment