PV finder - finding primary vertices in proton-proton collision
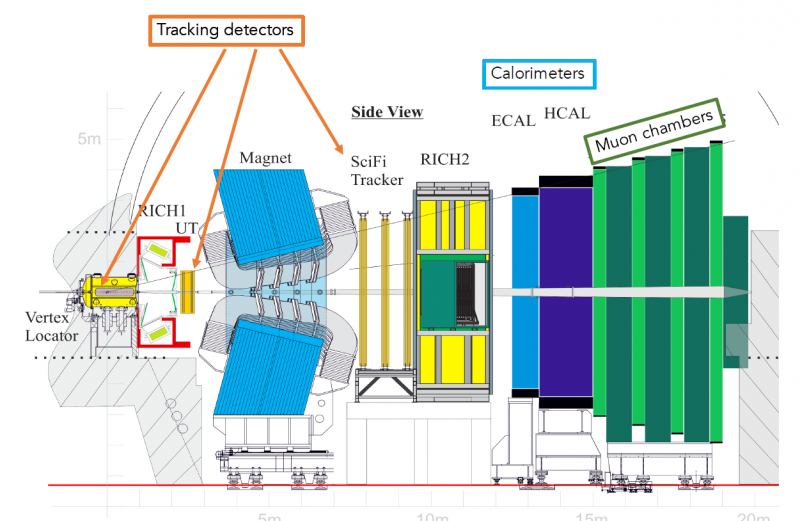
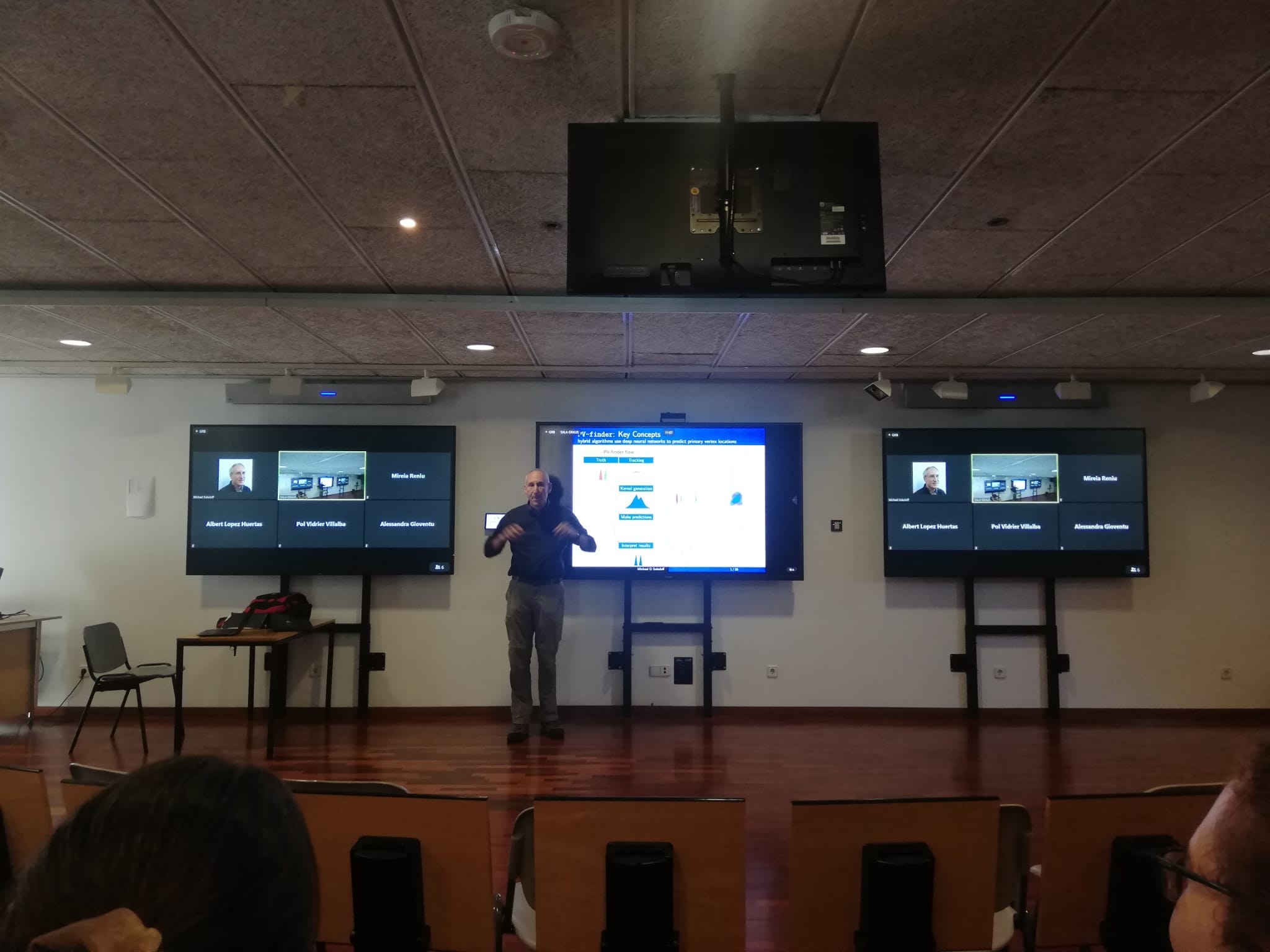
Talk by Dr. Mike Sokoloff organized by Dr. Xavier Vilasís where he reflects on his recent study.
We are studying the use of deep neural networks (DNNs) to identify and locate primary vertices (PVs) in proton-proton collisions at the LHC. Earlier work focused on finding primary vertices in simulated LHCb data using a hy- brid approach that started with kernel density estimators (KDEs) derived heuris- tically from the ensemble of charged track parameters and predicted “target his- togram” proxies, from which the actual PV positions are extracted. We have recently demonstrated that using a UNet architecture performs indistinguish- ably from a “flat” convolutional neural network model. We have developed an “end-to-end” tracks-to-hist DNN that predicts target histograms directly from track parameters using simulated LHCb data that provides better performance (a lower false positive rate for the same high efficiency) than the best KDE-to- hists model studied. This DNN also provides better efficiency than the default heuristic algorithm for the same low false positive rate. “Quantization” of this model, using FP16 rather than FP32 arithmetic, degrades its performance mini- mally. Reducing the number of UNet channels degrades performance more sub- stantially. We have demonstrated that the KDE-to-hists algorithm developed for LHCb data can be adapted to ATLAS and ACTS data using two variations of the UNet architecture. Within ATLAS/ACTS, these algorithms have been vali- dated against the standard vertex finder algorithm. Both variations produce PV- finding efficiencies similar to that of the standard algorithm and vertex-vertex separation resolutions that are significantly better.
Add new comment